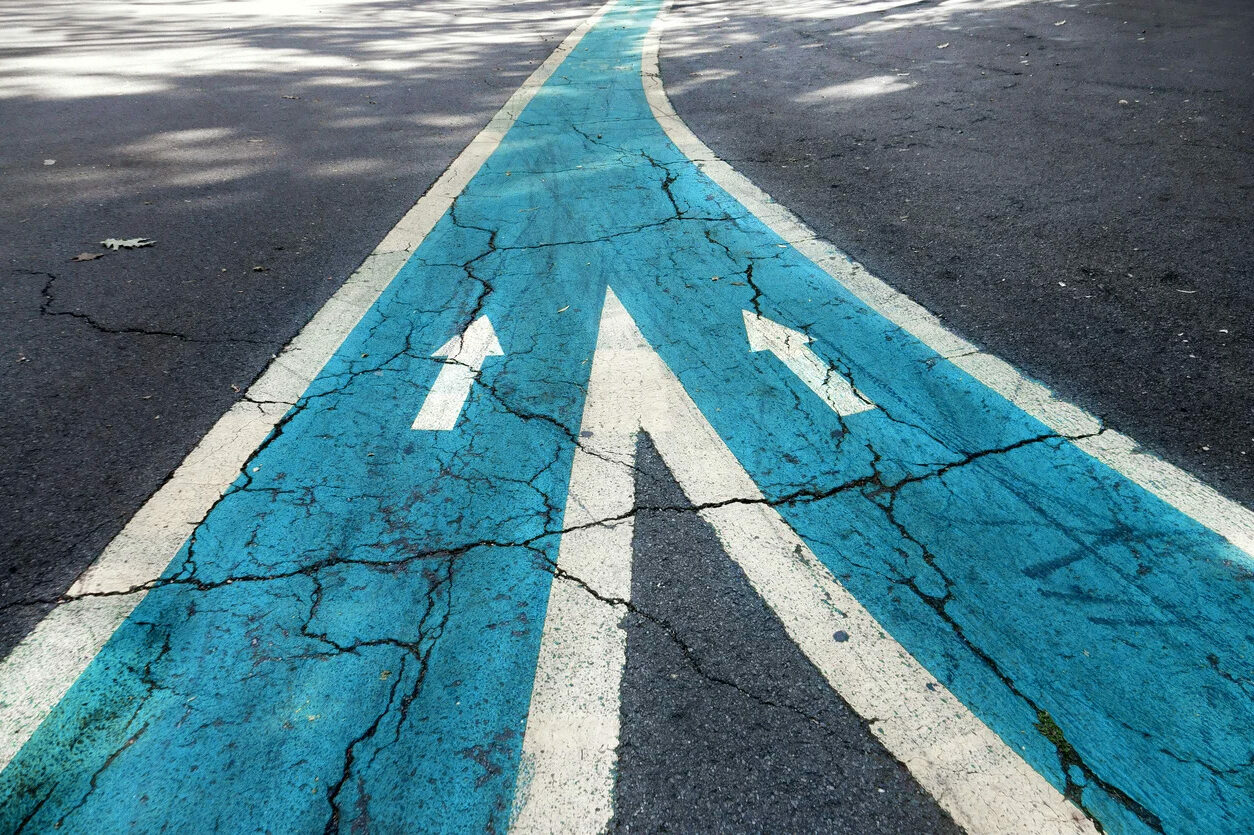
Forging a Path: Causal Inference and Data Science for Improved Policy
In the evolving landscape of statistical, econometric, and data science advancements, a significant number of innovative methodologies remain untapped by applied research. There is a disconnect between cutting-edge statistical tools and applied research questions addressing societies’ most pressing concerns.
This workshop seeks to address this gap and establish research collaboration between data scientists, experts in the causal inference literature, and applied researchers who better understand the empirical contexts, objectives, and challenges faced by policymakers. Our proposed program will facilitate a cross-disciplinary exchange, where applied researchers from different disciplines can present their research questions and methodological issues. In turn, both data science and causality researchers can guide discussions on new and existing methods while promoting their research agendas. Join us in this endeavour to foster collaborative exploration, amplifying the impact of causal inference and data science research on real-world policy challenges.
Funded through the DSI Emergent Data Sciences Program competition, and co-led by Professors Linbo Wang (Departments of Statistical Sciences and Computer Science), Gustavo J. Bobonis, Raji Jayaraman, and Ismael Mourifié (Department of Economics) at the University of Toronto.
Keynote Speakers
Alberto Abadie, Professor of Economics and Associate Director of the Institute for Data, Systems, and Society at MIT
Elizabeth Halloran, Professor, Vaccine and Infectious Diseases Division, Fred Hutchinson Cancer Center, and Department of Biostatistics, University of Washington